Christopher Manning, computer science expert and professor at Stanford University, discusses the development of AI and what the technology holds for the world of business
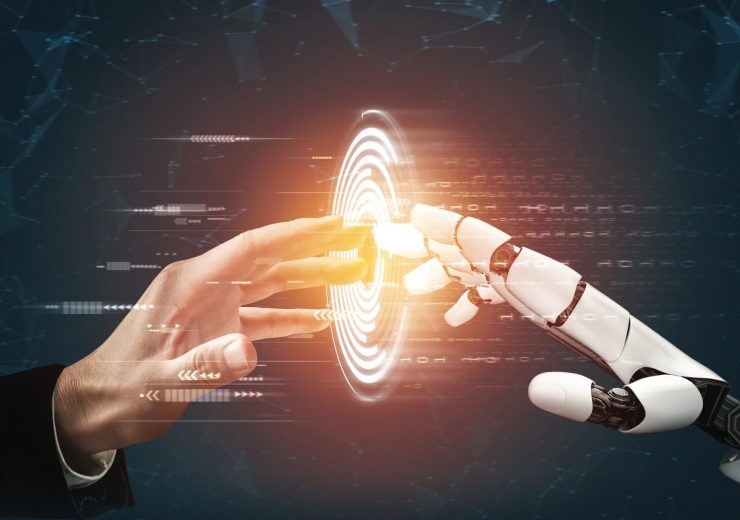
At a new age of AI, CEOs are desperately looking for people with a range of new digital skills (Credit: Blue Planet Studio/Shutterstock.com)
AI has been hailed as a game changer for the future of business. Christopher Manning, a renowned professor of computer science and linguistics at Stanford University – who advises Samsung electronics, among others – tells Stephen Hall how advanced AI could be set to change society.
For CEOs, the speed of change in the years leading up to 2020 has been unprecedented. The world is now standing on the precipice of a new age of artificial intelligence (AI) and it is at a crossroads that could go in a number of different directions.
“In short, the advent of super-intelligent AI would be either the best or the worst thing ever to happen to humanity,” said the late physicist, Stephen Hawking. “The real risk with AI isn’t malice, but competence. A super-intelligent AI will be extremely good at accomplishing its goals and if those goals aren’t aligned with ours, we’re in trouble.”
While machines surpassed human capabilities to calculate complicated mathematical equations and even dominated former world champion, Gary Kasparov, at chess decades ago, there have historically been noticeable gaps in their proficiency. Empathy, language acquisition and creativity have been listed as long-standing weaknesses, but we are now at a crucial juncture in this regard.
In the late 1800s, Alexander Graham Bell toiled in his studio to invent the telephone by experimenting with electrical systems to send audio data, before making a giant leap forward that revolutionised cross-border communication.
Fast forward to 2020, and the past few years have seen similar exponential strides onward in the field of AI.
Learning the language
Amid so much technological and societal transition, CEOs are desperately hunting for people with a range of new digital skills.
For Christopher Manning, professor of computer science and linguistics at Stanford University, the field of AI and machine language acquisition has been a topic he’s been engaged with for many years.
“It started right at the beginning of me being an undergraduate student,” he says. “I’d seen some of the ideas of linguistics, that there were these people who try and work out the structure of how languages work in general. So, you’re not just learning one particular language – like learning Spanish.
“That seemed like a cool idea to me, so from my first year at university I took computer science and linguistics. As an undergrad, they didn’t have any natural language processing or computational linguistics courses, so it wasn’t until later on, when I was a graduate student, that I started to do that.”
In terms of his influences, Manning has a strong linguistic education. He explains that this puts him at a distinct advantage, since most people who are involved in the study of natural language processing fall into it as computer scientists. This means that they don’t have any background on the topic.
Linguistics, as a subject – especially in the US – has historically been dominated by Noam Chomsky and Steven Pinker’s theories, who have argued that human languages aren’t learnable from the available data and, therefore, we have to assume innate language faculty in the brain.
“This has never seemed convincing to me,” says Manning. “And so, I’ve always been much more interested in machine-learning approaches, and how we can take data and discover linguistics structure. I think, not only are those kinds of approaches right, but they’ve become the main technological direction leading to the success of natural language processing systems that are being built.”
One of the future goals of AI researchers is the idea of machines thinking ‘like a child’, able to learn new things and getting better, and improving over time. Looking back at AI in the 1980s, the idea was that intelligent systems could be built by humans inputting rules and knowledge.
“What happened, starting in the 1990s and increasingly in the 21st century, is the idea that, actually, the way to make progress is to build learning systems so that they can gather and use data, extract information about how things work, generalise, get better over time and improve,” he explains.
“So, we certainly want things that are childlike, but another part of that is children seem to be messing around a lot, while they’re actually developing a sense of how the world works. Increasingly, in more sophisticated ways, they are coming to understand how things function.
“A lot of language understanding isn’t in terms of words, which have a past tense form or that you can put it together with some other word. It’s knowing how to interpret the vague, imprecise things we say in language and the way you do that is by having a good understanding of the world so you can interpret what people mean in context. Those are things we need to try and build into our artificial intelligence systems.”
Technology is developing fast
The world is in a period of rapid progress in this regard and a lot of this has been driven by exciting new developments in using large amounts of data, neural networks or deep-learning methods.
Manning has no doubt that this will continue and, he says, for the 25 or so years that he’s been involved in the field, the past five are by far the period of fastest development.
“There’s a genuine and real reason why there’s such a lot of excitement at the moment and I expect that will continue. We’ll start to have better learning methods, systems with more knowledge and understanding, showing broader intelligence rather than being able to do very specific and narrow tasks,” he explains.
“How far will that get us? It’s often hard to tell. In the 1960s, there were a lot of people who were predicting that we’d have human-level artificial intelligence within a decade. We didn’t even begin to get close to that.
“In a period of rapid progress, it’s really hard to tell whether that will continue and accelerate or whether you’ll hit a wall and need to wait until some fundamentally new ideas are found.”
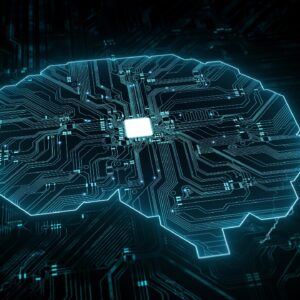
Looking ahead to the coming age of AI, Manning explains that he’s most excited about how machines can build up knowledge and use it for reasoning, which was the central part of AI thinking in the 1970s and 1980s.
Not very much progress was made but, when things really started to pick up steam in the 90s and 2000s, the initial focus was on more low-level signal processing tasks – things like computer vision object recognition and speech recognition.
This was good, in many ways, because it emphasised the use of machine-learning methods that people put on the shelf, including a lot of the questions about how people can build up knowledge and use it for reasoning. This, he points out, is very important for higher levels of AI, for planning and understanding the relationships between concepts.
The future of AI in business
Many people have said that they fear AI but, as Manning explains, some of this sensationalism is best ignored.
“There’s the one form of sensationalism where people are putting up pictures of science fiction robots with red eyes and arms with formidable weapons,” he says. “But I don’t think this is something that we genuinely need to be worried about.
“Arguably, that’s some kind of male fantasy rather than legitimate fears for the world but, on the other hand, there are real issues that are worth thinking about, which certainly include issues around job loss and labour markets.
“This is a complex subject for economists to think about, but my thoughts are it’s a process that has been going on for centuries. Once upon a time, 90% of people worked in agriculture and now that’s 2–3% and various other ways of employment have come along over time.
“It’s been the case that people have fixated on the jobs that are disappearing because it is very difficult to see the new opportunities that will be created, which will lead to new jobs.”
He gives the example of a bookkeeper, a job that diminished as people invented spreadsheets that could add up columns and numbers in no time at all. Once you could add up those columns and numbers, however, there were then numerous opportunities to get involved in financial modelling and understanding the effects of different decisions. This means far more people are employed using spreadsheets than were ever employed as bookkeepers.
“I think quite a few of the dangers come down to the fact that, on the one hand, the speed of technological change is increasing, whereas the speed of adaptability of human beings isn’t at the first approximation,” he concludes. “That’s clearly an issue with respect to social cohesion and social unrest. There are just lots of people who are middle-aged, who have a job they’ve always done and would like to keep doing that job.
“While there are certain things that governments and societies should be doing in terms of providing re-skilling opportunities and lifelong education, it is hard – people have a job, they don’t want to change and there’s no doubt there’s going to be a certain amount of pain as society adapts.
“If you’re an individual or a company, the advice is clearly the nimble, adaptable people – that can see opportunities and new ideas, and can move quickly into embracing new ways of working with artificial intelligence – are the people that are going to be the winners. Meanwhile, we also need to be supporting the people who aren’t thinking nimbly.”
This article first appeared in Chief Executive Officer magazine Vol 1 2020